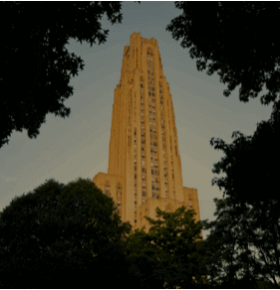
Subscribe to Pittwire Today
Get the most interesting and important stories from the University of Pittsburgh.Imagine this scenario: Someone feels slight chest pain and suspects a mild heart attack. They call 911 and are rushed to the hospital, where doctors and nurses spring into action, but it might be 24 hours before they know for sure whether a heart attack actually happened and how to treat it.
University of Pittsburgh School of Nursing researcher Salah Al-Zaiti is developing machine-learning technology to quickly detect a kind of heart attack that usually requires a prolonged battery of tests to identify.
These cases are distinct from massive heart attacks, where it’s obvious a major cardiac event has happened and the surgical and treatment paths are clear.
“Masked” heart attacks show symptoms ranging from tiredness to sweating that could be caused by a number of other ailments like muscle strain or the flu. Ambulance medics can administer an EKG on the way to the hospital, but with masked attacks, those results aren’t always enough to make a solid determination. In these situations, nurses and doctors may draw blood, run stress tests and CT scans, and observe while patients wait a day or more for results.
In the end, an average of 50% of these patients are sent home with a non-heart attack diagnosis — but the process is inefficient, costly and frustrating for everyone. Al-Zaiti’s project shortens that identification process through a computer program that deepens the analysis of the EKG’s waveform measure of heart activity and gives results in real time.
“We want to give doctors a quicker path to certainty and reassure patients early,” said Al-Zaiti. Since 2015, his team has recruited more than 4,000 study participants, and in testing the technology in Pittsburgh ambulances, they’ve achieved 80% accuracy in determining whether a patient has had a heart attack or not before arriving at the hospital.
Now he’s advancing the technology further, refining artificial intelligence algorithms to pinpoint where the problem lies in those 10% of patients who had a masked heart attack, exposing exactly which artery was blocked and by how much. Finding the blockage is key to determining treatment, but it can be difficult to isolate the location of the blockage in masked attacks using traditional tools.
Al Zaiti’s new web-based program will configure many data points and produce graphs of various EKG markers and a diagram of the coronary arteries, with each heart valve labeled “likely” or “unlikely” for occlusions. It also calculates the likelihood of a major adverse cardiac event in the 30-day period following admission, a key factor in deciding how aggressively to treat a heart problem. Data can be transmitted from the EKG — from inside the hospital or from an ambulance out on a call — to the physician’s computer in about 60 seconds.
To develop the program, Al-Zaiti assembled an interdisciplinary team of Pitt researchers, among them Ervin Sejdic from the Swanson School of Engineering, as well as Samir Saba from cardiology, and Clifton Callaway and Christian Martin-Gill from emergency medicine, all in the School of Medicine. Funded by the National Institutes of Health, the program is being tested in Pittsburgh now in “silent mode,” with results shown only to Al-Zaiti’s team, but he hopes it will be approved for use in hospitals throughout the country in the future.
“Lots of studies come up with ideas for fancy solutions to problems but that’s it,” Al-Zaiti said. “We want to take the next step of implementation so it can be used to improve patient care.”
Al-Zaiti has received wide recognition for his novel developments and research, including two American Heart Association Early Career Investigator Awards, and in 2017, he was inducted as a fellow of the Council on Cardiovascular and Stroke Nursing.
This story appears in the latest Pitt Nurse magazine, which will be hitting mailboxes soon.