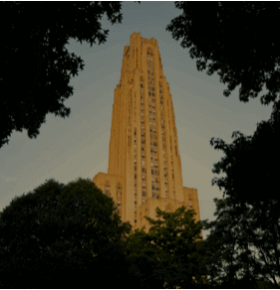
Subscribe to Pittwire Today
Get the most interesting and important stories from the University of Pittsburgh.Signal from Noise: Math Professor Wins Fellowship to Delve Into the Noisy Brain
The brain can be a pretty noisy place.
People often think of the brain as an orderly machine that functions akin to a computer. But when scientists measure brain activity while a person performs a task, they also find substantial, seemingly random background “noise” in their measurements.
Is this noise harmless interference? Is it inhibiting the brain’s ability to learn?
“Not only are these fluctuations not destructive, they stabilize network-wide learning,” said Brent Doiron, professor of mathematics and member of the joint University of Pittsburgh-Carnegie Mellon University Center for the Neural Basis of Cognition.
Throughout his time at Pitt, Doiron has become an emerging force in the area of theoretical neuroscience — a field that seeks to build mathematical and computation models of the brain — rather than using MRIs or other imaging techniques — to figure out how our brains work. His central challenge? Understanding all that noise.
Doiron’s theoretical work involves both pad-and-paper mathematics as well as large-scale computer simulations of brain activity. His model considers brain-based measurements such as the distance between neurons as well as the timing of their activity after stimulus to understand how “noise” is distributed over space and time in brain tissue.
“Brain activity looks noisy, but in our models it’s really a replay of past experiences,” Doiron explained. In other words, he believes that the brain isn’t solely focused on reading the text on this screen. It’s doing unrelated background thinking, too.
Recently, Doiron, who’s also part of Pitt’s Center for Neuroscience and Pitt’s Brain Institute, and colleagues examined experimental data and found that their model accurately predicted how neurons would act based on how far apart they were from one another. The model, Doiron said, could help researchers better understand how the brain computes information because it helps to describe how neural network structure relates to how neurons behave within a given network. And, as the saying goes, form follows function.
Doiron is getting a big chance to test and further refine this theory when his five-year, $2.2 million U.S. Department of Defense Vannevar Bush Faculty Fellowship kicks in this September. The program provides research funding to conduct revolutionary “high-risk, high-payoff” research of strategic importance to the Department of Defense (DoD).
Doiron is one of only 13 distinguished university faculty scientists and engineers across the United States that make up the 2017 class of Vannevar Bush Faculty Fellows. Present and past fellows are among the nation’s best scientists, with many members of the National Academy of Sciences and even a Nobel laureate on the list.
In the case of Doiron’s work, the DoD hopes to someday apply his findings to understanding post-traumatic stress syndrome — a condition in which Doiron believes the brain can’t sort signal from noise — and to enhance learning in stressful situations. This will require understanding not just how neurons connect, but how those connections connect into a larger network. The Vannevar Bush fellowship will help him break into this field.
It’s not all math and models, however. Theories of brain computation should also include biological realism. To tackle how noise interacts with learning, Doiron will team up with Pitt assistant professor of neuroscience Anne-Marie Oswald, who is also his wife.
Oswald’s lab trains mice to make decisions based on their powerful sense of smell and then identifies and manipulates the networks of neurons involved.
“The sense of smell and associative memory are deeply linked. Experience an aroma from childhood and a flood of other memories come to the surface,” Oswald said.
Using powerful genetic models, her lab can dissect how odors are stored in the brain, exactly the kind of experiments that can really test the biology behind Doiron’s models.
“This back-and-forth between experiment and theory pushes both fields. If we want to understand the brain, more and more teams will need to follow this model,” Doiron said.