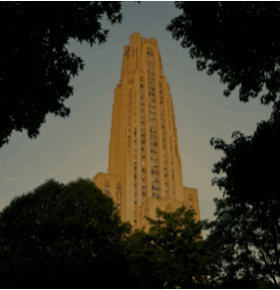
Subscribe to Pittwire Today
Get the most interesting and important stories from the University of Pittsburgh.The United States may have experienced an overall slight decrease in overdose deaths from prescription drugs between 2016 and 2017, but researchers aren’t taking a break from trying to eradicate this ongoing problem.
Anyone can become addicted to opioid medication, but figuring out who is most at risk for overdose can be tricky.
“We all want to identify high-risk patients, but no one really knows how to do that well,” said Walid Gellad, associate professor of medicine and health policy at the University of Pittsburgh and director of Pitt’s Center for Pharmaceutical Policy and Prescribing.
Gellad is teaming up with Allegheny County officials and national health care databanks in two separate studies on the local and national levels to better identify at-risk individuals for opioid overdose using machine learning, a branch of artificial intelligence where computing systems can make decisions with minimal human intervention by identifying patterns and interpreting data.
Gellad has found that machine-learning algorithms — processes computers must follow for calculations — perform well for risk prediction and stratification of opioid overdose risk, especially in identifying subgroups that have minimal risk of overdose.
For example, one of his team’s algorithms was able to identify a subset of people who use Medicare as a low-risk group, in which only one in 10,000 had an overdose episode.
“Groups with low risk may potentially be excluded from interventions or requirements that place a burden on them, because of this low risk,” Gellad said.
Gellad added that the team is applying this algorithm to people who just started on a prescription for opioids. They will then examine what their risk is over the next three months for an overdose.
“Conventional wisdom would say that someone who fills a really high-dose prescription is at a higher risk for overdose. But with machine learning, we see more details about that person that could impact their risk, such as someone who perhaps has a high dose combined with a history of overdose, who got out of jail recently, and has a psychiatric disorder or other serious health condition that is being treated,” he said.
In a study published this March, Gellad and his team predicted opioid overdose among national Medicare beneficiaries and Pennsylvania Medicaid beneficiaries. This study is funded by the National Institutes of Health and the National Institute on Drug Abuse.
Gellad, along with collaborators from Pitt, the University of Florida and University of Utah, examined the health data on more than a half-million Medicare beneficiaries who filled one or more prescriptions for opioids between 2011 and 2015. They found that more than 90% of Medicare beneficiaries in a cohort filling prescription opioids have little to no risk of overdosing.
This approach may not only potentially save lives, but also save money. The annual cost of misuse or abuse of opioids exceeds $78.5 billion, including the costs of health care, lost productivity, substance abuse treatment and the criminal justice system, according to the study.
For the ongoing county study, which uses machine learning like the national study, Gellad is teaming up with the Allegheny County Department of Human Services to work with its Allegheny County Data Warehouse to complete similar analyses.
Despite its promising results, the AI approach does have some limitations, mainly in terms of qualitative research.
“People are complex and they have many factors in their lives,” said Eric Hulsey, manager of behavioral health analytics at the Allegheny County Department of Human Services. “For example, we don’t know their stories or how they use drugs or when they started using them.”
Hulsey is also an adjunct professor of behavioral and community health sciences in Pitt’s Graduate School of Public Health.
“We’ve performed analytics work on this before for opioid hot spots and have partnered with the Allegheny County Health Department, local law enforcement and the county jail to better identify opportunities for intervention, such as targeted priority distribution of naloxone,” Hulsey said. “But here, we wanted to go deeper than just population areas. We wanted to identify individual risk factors.”
This approach may not only potentially save lives, but also save money. The annual cost of misuse or abuse of opioids exceeds $78.5 billion, including the costs of health care, lost productivity, substance abuse treatment and the criminal justice system, according to the study.
“We don’t have unlimited money and time, so it’s better to take the resources we have and identify the high-risk populations rather than everyone,” Gellad said.
The county study is partially funded by a two-year grant from the Richard King Mellon Foundation, which will continue to support the effort through 2019.